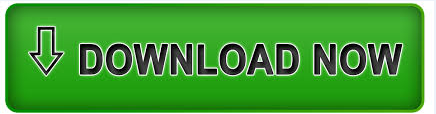
The similarities between the process of developing scientific theories and the way that children construct an understanding of the world around them have led to the child-as-scientist metaphor in developmental psychology, which views conceptual changes during development as a form of scientific theory discovery 4, 5. This faculty is most clearly manifested in the historical development of science 1 but also occurs in miniature in everyday cognition 2 and during childhood development 3. These results suggest routes to more powerful machine-enabled discovery of interpretable models in linguistics and other scientific domains.Ī key aspect of human intelligence is our ability to build theories about the world. Finally, the same algorithm captures few-shot learning dynamics, acquiring new morphophonological rules from just one or a few examples. Joint inference across all 70 data sets automatically synthesizes a meta-model encoding interpretable cross-language typological tendencies. Across 70 datasets from 58 diverse languages, our system synthesizes human-interpretable models for core aspects of each language’s morpho-phonology, sometimes approaching models posited by human linguists.

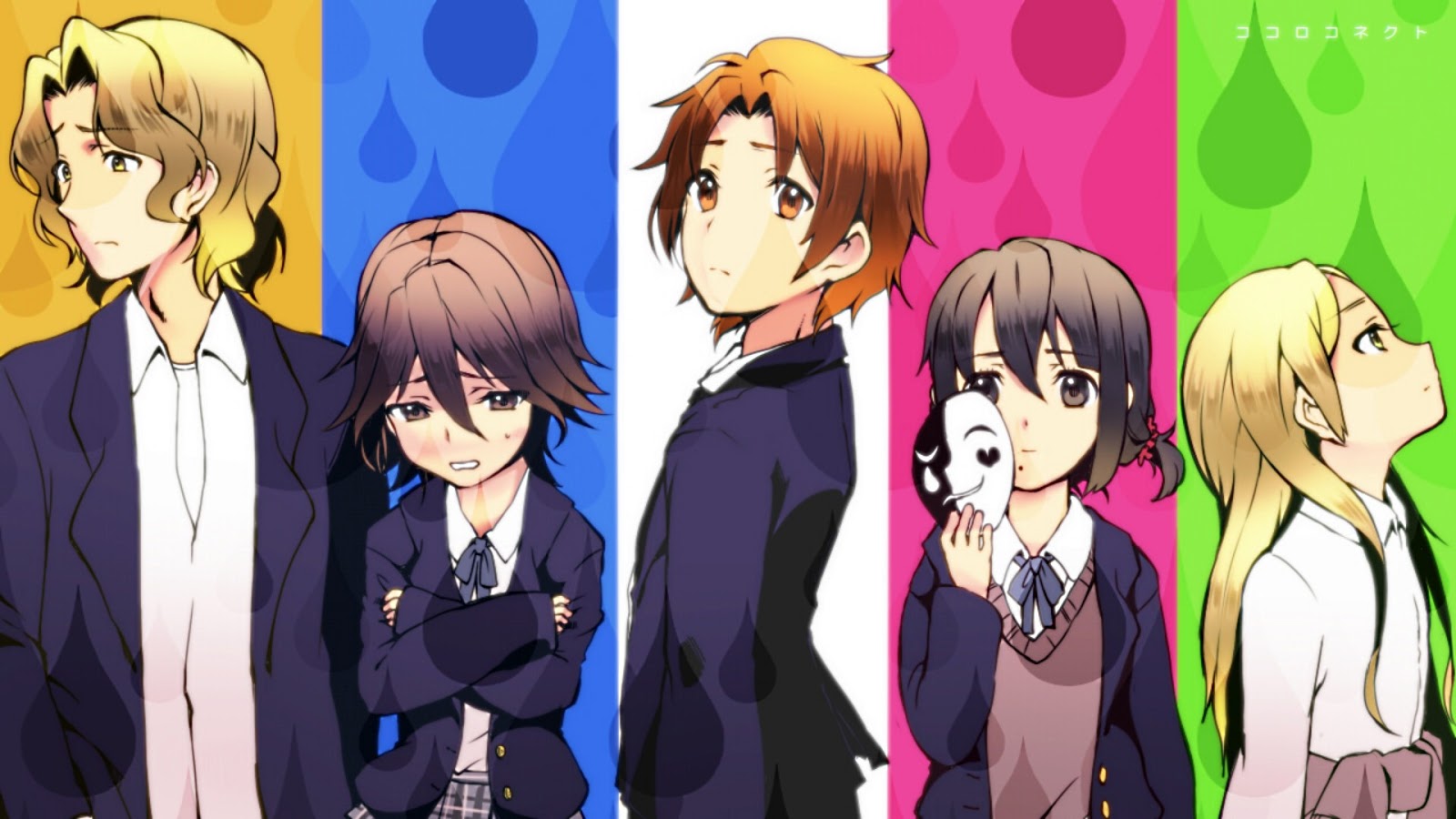
We integrate Bayesian inference with program synthesis and representations inspired by linguistic theory and cognitive models of learning and discovery. We present a framework for algorithmically synthesizing models of a basic part of human language: morpho-phonology, the system that builds word forms from sounds. Automated, data-driven construction and evaluation of scientific models and theories is a long-standing challenge in artificial intelligence.
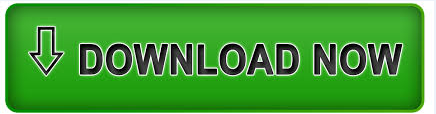